If AI is a Body, the Data That Powers it is the Blood

Author:
Michael Mbagwu, MD
For anyone who has followed the news around ChatGPT and its creator OpenAI, the idea of widespread application of artificial intelligence (AI) in healthcare could be a cause for concern. The technology, which garnered considerable attention in the tech industry following its introduction a year ago, has seemingly astounded observers with its remarkable capabilities to ace the bar exam and communicate in a manner that is indistinguishable from real human speech. It also introduced many concerns involving potential misuses and other risks.
In healthcare, these concerns have been amplified by the fact that some AI solutions in the past have over-promised and under-delivered, and most of us find it hard to imagine a world where the clinical areas physicians have cultivated expertise over the course of decades could be replicated by an algorithm. However, despite some healthy skepticism, AI has quietly revolutionized certain aspects of healthcare research and analysis using real-world evidence (RWE) to surface insights in seconds, which would have taken months or more to produce a few years ago. The key to separating the substance from the hype is to focus less on the ostentatious new AI tools making headlines and more on the underlying data that is being used to develop and train large language models for specialized healthcare tasks.
Just as anyone who is mindful about what goes into their body should read the Nutrition Facts label on the foods they buy before they consume them, anyone using AI in healthcare should follow the same good sense when it comes to the data that powers our algorithms.
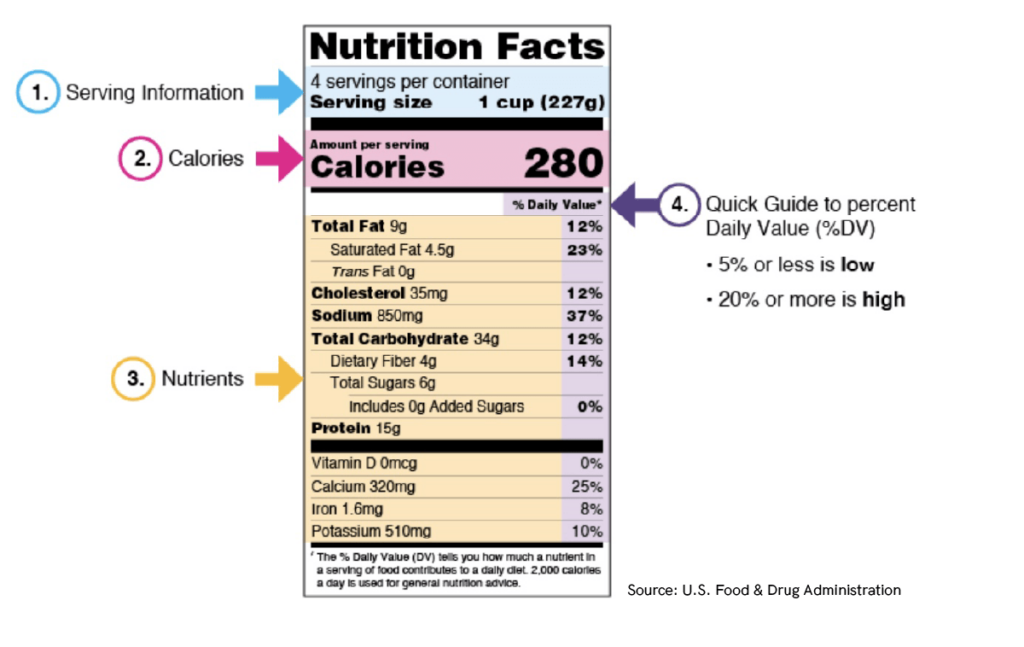
Focusing on Data to Address Bias in AI Models
This is where healthcare presents some unique challenges. The underlying data typically comes from highly disparate sources: medical claims data, electronic health record (EHR) data, lab results, and other structured and unstructured information. As one might expect, this is notoriously challenging to work with. It is not the kind of publicly available, black-and-white data that ChatGPT used to pass a multiple choice exam. It is hugely specialized, infinitely nuanced and requires expert human intervention before it can be built into an algorithm.
In my field of ophthalmology, for example, optical coherence tomography (OCT) is a common imaging modality which uses light waves to take cross-section pictures of the optic nerve and retina. This allows ophthalmologists to map and measure thickness and diagnose certain conditions. Results are captured from each eye and compared to a normative database, which establishes the benchmark for “normal” retinal nerve fiber layer or macular thickness. However, recent studies have found that OCT normative databases, often consisting of data sourced from predominantly Caucasian patients, may not accurately capture normal anatomic variations unique to Black or Asian patient populations.
In practical terms, algorithms based on non-diverse patient data may result in erroneous conclusions. And, consequently, delayed or missed diagnoses.
A related phenomenon can occur when researchers attempt to build a comprehensive algorithm based only on a subset of available clinical information. For instance, claims data that is only capturing structured diagnostic codes (i.e. International Classification of Diseases/ICD-10), but not capturing clinical notes and other unstructured details. We see this frequently in neurology where important clinical details, such as multiple sclerosis subtype, or the cardinal signs of Parkinson’s Disease, are not captured in many off-the-shelf datasets because they can only be found by parsing and curating data from clinical notes.
Setting the Standard for AI in Healthcare
In both scenarios mentioned above, a predictive model attempting to tackle a broad question but grounded in a limited dataset will consistently arrive at inaccurate conclusions. All due to lack of the necessary information. Paying meticulous attention to those details and identifying anomalies where they lie is at the very heart of research-grade data science. That same rigor should be applied to AI models being used in healthcare.
The good news is that the technology—and the computing power necessary to use it—is more accessible than ever. Pioneers in data harmonization are already doing the hard work necessary to incorporate race and ethnicity data, unstructured data from clinical notes and other details that ensure analytic models drive useful insights without perpetuating disparities. We are living in a truly exciting moment where decisions made today will influence how we diagnose and treat diseases in the future. Let’s do it the right way.

Let's Accelerate Research Together
To learn more about Verana Health, please fill out the information below and our team will follow up with you as soon as possible.